This page is a rebuild of the original page, which can be found here
Overview
Welcome to join our ICCV 2023 Workshop!The Workshop on Observing and Understanding Hands in Action (HANDS) will gather vision researchers working on perceiving hands performing actions, including 2D & 3D hand detection, segmentation, pose/shape estimation, tracking, etc. The seventh edition of this workshop (HANDS@ICCV2023) will emphasize hand pose estimation from the egocentric view and hands performing fine-grained actions and interactions with tools and objects.
Development of RGB-D sensors and camera miniaturization (wearable cameras, smart phones, ubiquitous computing) have opened the door to a whole new range of technologies and applications which require detecting hands and recognizing hand poses in a variety of scenarios, including AR/VR, assistive systems, robot grasping, and health care. However, the tasks of hand pose estimation from an egocentric camera and/or in the presence of heavy occlusion are still challenging under the status quo.
Compared to static camera settings, recognizing hands in egocentric images is a more difficult problem due to viewpoint bias, camera distortion (e.g., fisheye), and motion blur from the head movement. Additionally, addressing the occlusion during hand-object or hand-hand interactions is an important open challenge that still attracts significant attention for real-world applications. We will also cover related applications, including gesture recognition, hand-object manipulation analysis, hand activity understanding, and interactive interfaces. The relevant topics include:
Topics
We will cover all hand-related topics. The relevant topics include and not limited to:- 2D/3D hand pose estimation
- Hand shape estimation
- Hand-object/hand interaction
- Hand detection/segmentation
- Gesture recognition/interfaces
- 3D hand tracking and motion capture
- Hand modeling and rendering
- Egocentric vision
- Hand activity understanding
- Robot grasping and object manipulation
- Hand image capture and camera systems
- Efficient hand annotation methods and devices
- Algorithm, theory, and network architecture
- Efficient learning methods with limited labels
- Generalization and adaptation to unseen users and environments
- Applications in AR/VR, Robotics, and Haptics
Schedule(Paris Time)
Monday afternoon (13:30-17:30), October. 2. 2023 W5, Paris Convention Center, France
13:30 - 13:40 | Opening Remarks |
13:40 - 14:10 | Invited Talk: He Wang |
Title: Learning universal dexterous grasping policy from 3D point cloud observations Abstract: Dexterous hand grasping is an essential research problem for vision, graphics, and robotics communities. In this talk, I would first cover our recent work, DexGraspNet, on synthesizing million-scale diverse dexterous hand grasping data, which won ICRA 2023 outstanding manipulation paper award finalist. Based on this data, our CVPR 2023 work, UniDexGrasp, learns a generalizable point cloud based dexterous grasping policy that can generalize across thousands of objects. We further extend this work to UniDexGrasp++, accepted as an ICCV oral, that proposes a general framework that greatly enhances the success rate to more than 80%. | |
|
|
14:10 - 14:40 | Invited Talk: Gül Varol |
Title: Automatic annotation of open-vocabulary sign language videos Abstract: Research on sign language technologies has suffered from the lack of data to train machine learning models. This talk will describe our recent efforts on scalable approaches to automatically annotate continuous sign language videos with the goal of building a large-scale dataset. In particular, we leverage weakly-aligned subtitles from sign interpreted broadcast footage. These subtitles provide us candidate keywords to search and localise individual signs. To this end, we develop several sign spotting techniques: (i) using mouthing cues at the lip region, (ii) looking up videos from sign language dictionaries, and (iii) exploring the sign localisation that emerges from the attention mechanism of a sequence prediction model. We further tackle the subtitle alignment problem to improve their synchronization with signing. With these methods, we build the BBC-Oxford British Sign Language Dataset (BOBSL), continuous signing videos of more than a thousand hours, containing millions of sign instance annotations from a large vocabulary. These annotations allow us to train large-vocabulary continuous sign language recognition (transcription of each sign), as well as subtitle-video retrieval, which we hope will open up new possibilities towards addressing the currently unsolved problem of sign language translation in the wild. | |
|
|
14:40 - 15:10 | Invited Talk: Gyeongsik Moon |
Title: Towards 3D Interacting Hands Recovery in the Wild Abstract: Understanding interactions between two hands is critical for analyzing various hand-driven social signals and the manipulation of objects using both hands. Recently introduced large-scale InterHand2.6M dataset enabled learning-based approaches to recover 3D interacting hands from a single image. Despite the significant improvements, most methods have focused on recovering 3D interacting hands mainly from images of InterHand2.6M, which have very different image appearances compared to those of in-the-wild images as it was captured in a constraint studio. For the 3D interacting hands recovery in the wild, this talk will introduce two recent works: one for the algorithmic approach and the other for the dataset approach where each is accepted by CVPR 2023 and NeurIPS 2023. For the algorithmic approach, we introduce InterWild, a 3D interacting hands recovery system that brings inputs from in-the-lab and in-the-wild datasets to a shared domain to reduce the domain gap between them. For the dataset approach, we introduce our new dataset, Re:InterHand, which consists of accurately tracked 3D geometry of interacting hands and rendered images with a pre-trained state-of-the-art relighting network. As the images are rendered with lighting from high-resolution environment maps, our Re:InterHand dataset provides images with highly diverse and realistic appearances. As a result, 3D interacting hands recovery systems trained on Re:InterHand achieve better generalizability to in-the-wild images than simply training it on in-the-lab datasets. | |
|
|
15:10 - 16:10 | Poster List Coffee break time & Poster |
16:10 - 16:40 | Invited Talk: David Fouhey |
Title: From Hands In Action to Possibilities of Interaction Abstract: In this talk, I'll show some recent work from our research group spanning the gamut from understanding hands in action to imagining possibilities for interaction. In the first part, I'll focus on a new system and dataset for obtaining a deeper basic understanding of hands and in-contact objects, including tool use. The second part looks forward towards the future and will show a new system that aims to provide information at potential interaction sites. | |
|
|
16:40 - 17:10 | Invited Talk: Lixin Yang |
Title: Paving the way for further understanding in human interactions with objects in task completion: the OakInk and OakInk2 datasets Abstract: Researching how humans accomplish daily tasks through object manipulation presents a long-standing challenge. Recognizing object affordances and learning human interactions with these affordances offers a potential solution. In 2022, to facilitate data-driven learning methodologies, we proposed OakInk, a substantial knowledge repository consisting of two wings: 'Oak' for object affordances and 'Ink' for intention-oriented, affordance-aware interactions.This talk will introduce our work in 2023: we expanded the OakInk methodology, giving rise to OakInk2 - a comprehensive dataset encompassing embodied hand-object interactions during complex, long-horizon task completion. OakInk2 incorporates demonstrations of 'Primitive Tasks', defined as minimal interactions necessary for fulfilling object affordance attributes, and 'Combined Tasks', which merge Primitive Tasks with specific dependencies. Both OakInk and OakInk2 capture multi-view image streams, provide detailed pose annotations for embodied hands and diverse interacting objects, and scrutinize dependencies between Primitive Task completion and underlying object affordance fulfillment. With all these knowledge incoporated, we show that OakInk and OakInk2 will provide strong support for a variety of tasks including hand-object reconstruction, motion synthesis, and the planning, imitation, and manipulation within the scope of embodied AI. | |
|
|
17:10 - 17:17 | Report: Aditya Prakash |
Title: Reducing Scale Ambiguity due to Data Augmentation | |
17:17 - 17:24 | Report: Karim Abou Zeid |
Title: Joint Transformer | |
17:24 - 17:31 | Report: Zhishan Zhou |
Title: A Concise Pipeline for Egocentric Hand Pose Reconstruction | |
17:31 - 17:31 | Closing Remarks |
Accepted Papers & Extended Abstracts
We are delighted to announce the following accepted papers and extended abstracts will appear in the workshop! All Extended abstracts and invited posters should prepare posters for communication during the workshop.
Poster size: the posters should be portrait (vertical), with a maximum size of 90x180 cm.
Accepted Extended Abstracts
- OakInk2 : A Dataset for Long-Horizon Hand-Object Interaction and Complex Manipulation Task
Completion.
Xinyu Zhan*, Lixin Yang*, Kangrui Mao, Hanlin Xu, Yifei Zhao, Zenan Lin, Kailin Li, Cewu Lu.
[pdf]
- A Novel Framework for Generating In-the-Wild 3D Hand Datasets.
Junho Park*, Kyeongbo Kong*, Suk-ju Kang.
[pdf]
- New keypoint-based approach for recognising British Sign Language (BSL) from sequences.
Oishi Deb*, Prajwal KR, Andrew Zisserman.
[pdf]
- Text-to-Hand-Image Generation Using Pose- and Mesh-Guided Diffusion.
Supreeth Narasimhaswamy, Uttaran Bhattacharya, Xiang Chen, Ishita Dasgupta, and Saayan Mitra.
[pdf]
- Hand Segmentation with Fine-tuned Deep CNN in Egocentric Videos.
Eyitomilayo Yemisi Babatope, Alejandro A. Ramírez-Acosta, Mireya S. García-Vázquez.
[pdf]
Technical Reports
- A Concise Pipeline for Egocentric Hand Pose Reconstruction.
Zhishan Zhou*, Zhi Lv*, Shihao Zhou, Minqiang Zou, Tong Wu, Mochen Yu, Yao Tang, Jiajun Liang.
[pdf]
- Multi-View Fusion Strategy for Egocentric 3D Hand Pose Estimation.
Zhong Gao, Xuanyang Zhang.
[pdf]
- Egocentric 3D Hand Pose Estimation.
Xue Zhang, Jingyi Wang, Fei Li, Rujie Liu.
[pdf]
- Reducing Scale Ambiguity due to Data Augmentation in 3D Hand-Object Pose Estimation.
Aditya Prakash, Saurabh Gupta.
[pdf]
Invited Posters
- Spectral Graph-Based Transformer for Egocentric Two-Hand Reconstruction using Multi-View Color
Images.
Tze Ho Elden Tse, Franziska Mueller, Zhengyang Shen, Danhang Tang, Thabo Beeler, Mingsong Dou, Yinda Zhang, Sasa Petrovic, Hyung Jin Chang, Jonathan Taylor, Bardia Doosti.
[pdf] [supp]
- Deformer: Dynamic Fusion Transformer for Robust Hand Pose Estimation.
Qichen Fu, Xingyu Liu, Ran Xu, Juan Carlos Niebles, Kris M. Kitani.
[pdf] [supp]
- HandR2N2: Iterative 3D Hand Pose Estimation Using a Residual Recurrent Neural Network.
Wencan Cheng, Jong Hwan Ko.
[pdf]
- HoloAssist: an Egocentric Human Interaction Dataset for Interactive AI Assistants in the Real
World.
Xin Wang, Taein Kwon, Mahdi Rad, Bowen Pan, Ishani Chakraborty, Sean Andrist, Dan Bohus, Ashley Feniello, Bugra Tekin, Felipe Vieira Frujeri, Neel Joshi, Marc Pollefeys.
[pdf] [supp]
- MHEntropy: Entropy Meets Multiple Hypotheses for Pose and Shape Recovery.
Rongyu Chen, Linlin Yang, Angela Yao.
[pdf] [supp]
- UniDexGrasp++: Improving Dexterous Grasping Policy Learning via Geometry-Aware Curriculum and
Iterative Generalist-Specialist Learning.
Weikang Wan, Haoran Geng, Yun Liu, Zikang Shan, Yaodong Yang, Li Yi, He Wang.
[pdf] [supp]
- Decoupled Iterative Refinement Framework for Interacting Hands Reconstruction from a Single
RGB Image.
Pengfei Ren, Chao Wen, Xiaozheng Zheng, Zhou Xue, Haifeng Sun, Qi Qi, Jingyu Wang, Jianxin Liao.
[pdf] [supp]
- HaMuCo: Hand Pose Estimation via Multiview Collaborative Self-Supervised Learning.
Xiaozheng Zheng, Chao Wen, Zhou Xue, Pengfei Ren, Jingyu Wang.
[pdf] [supp]
- Realistic Full-Body Tracking from Sparse Observations via Joint-Level Modeling.
Xiaozheng Zheng, Zhuo Su, Chao Wen, Zhou Xue, Xiaojie Jin.
[pdf] [supp]
- CHORD: Category-level Hand-held Object Reconstruction via Shape Deformation.
Kailin Li, Lixin Yang, Haoyu Zhen, Zenan Lin, Xinyu Zhan, Licheng Zhong, Jian Xu, Kejian Wu, Cewu Lu.
[pdf] [supp]
- OCHID-Fi: Occlusion-Robust Hand Pose Estimation in 3D via RF-Vision.
Shujie Zhang, Tianyue Zheng, Zhe Chen, Jingzhi Hu, Abdelwahed Khamis, Jiajun Liu, Jun Luo.
[pdf]
- Multimodal Distillation for Egocentric Action Recognition.
Gorjan Radevski, Dusan Grujicic, Matthew Blaschko, Marie-Francine Moens, Tinne Tuytelaars.
[pdf] [supp]
- FineDance: A Fine-grained Choreography Dataset for 3D Full Body Dance Generation.
Ronghui Li, Junfan Zhao, Yachao Zhang, Mingyang Su, Zeping Ren, Han Zhang, Yansong Tang, Xiu Li.
[pdf] [supp]
Invited Speakers
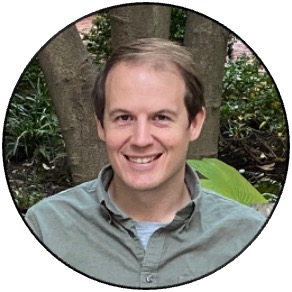
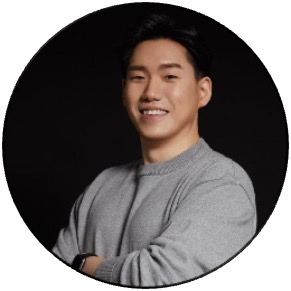
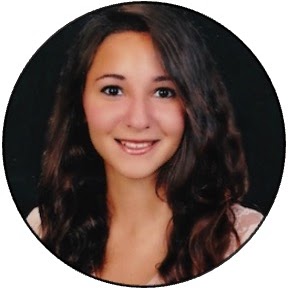
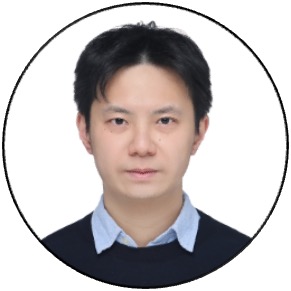
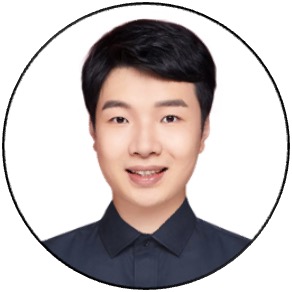
Organizers
Sponsors
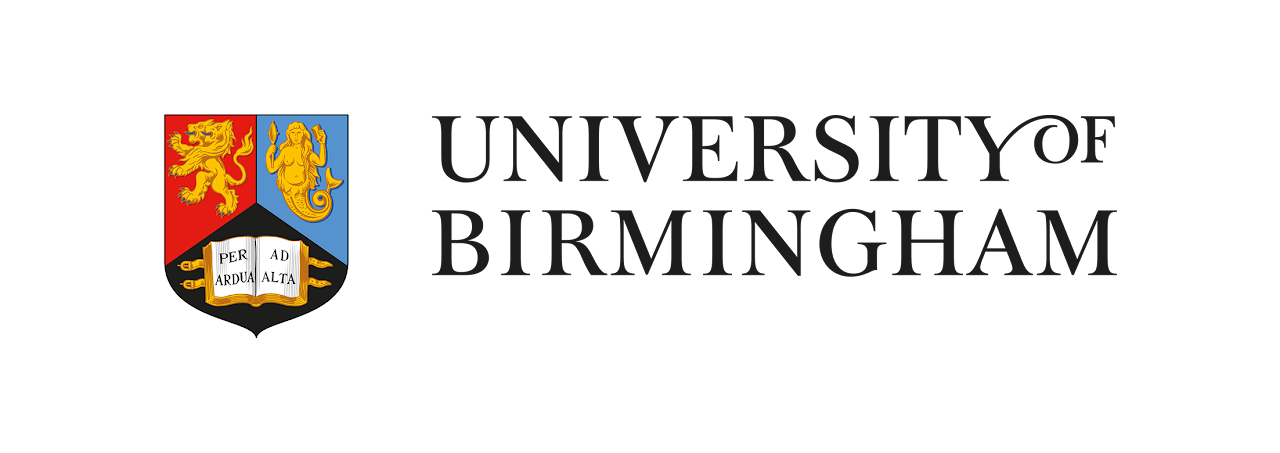
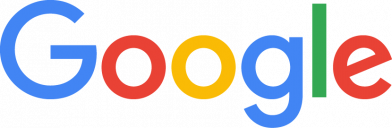
Contact
hands2023@googlegroups.com